In the rapidly maturing landscape of artificial intelligence (AI), multimodal learning has emerged as a game-changer. It enables AI systems to process and integrate data from numerous modalities, such as text, images, audio, and video. This approach is crucial for developing AI systems that can understand and interact with the world in a more human-like manner, as our experiences and communication are inherently multimodal.
However, traditional language models, trained solely on textual data, face limitations in capturing and representing the rich and diverse knowledge in various modalities. This is where the concept of Multimodal Retrieval Augmented Generation (MM-RAG) comes into play, offering a powerful solution to enhance the capabilities of AI systems.
The Need for External Knowledge Integration
One of the critical challenges faced by AI systems, particularly language models, is the ability to effectively capture and represent the vast amount of world knowledge. While large language models like GPT-4 and Llama-2 have demonstrated impressive capabilities in understanding and generating human-like text, they often need help with factual accuracy, hallucination, and incorporating domain-specific or rapidly changing information.
Researchers have recognized the importance of incorporating external knowledge sources into AI systems to address this challenge. By integrating external knowledge, these systems can access up-to-date and reliable information, reducing the risk of generating inaccurate or misleading outputs.
Retrieval Augmented Generation (RAG)
What is RAG?
Retrieval Augmented Generation (RAG) is a technique that combines the power of large language models with information retrieval capabilities. In this approach, a language model is augmented with a retrieval component that can access external knowledge sources, such as documents, databases, or web pages.
The retrieval component searches for relevant information from external knowledge sources based on the input prompt or context during the generation process. This retrieved information is used as additional context to inform and guide the language model's generation process, resulting in more accurate, diverse, and reliable outputs.
Benefits of RAG
The incorporation of RAG into language models offers several benefits:
1. Improved accuracy and reduced hallucination: By leveraging external knowledge sources, RAG helps language models ground their outputs in factual information, reducing the risk of generating plausible-sounding but factually incorrect content.
2. Incorporation of external knowledge sources: RAG enables language models to access and utilize a wide range of external knowledge sources, including domain-specific information, rapidly changing data, and specialized knowledge bases.
3. Transparency and verifiability: RAG provides users with insights into the sources of information used by the language model, enhancing transparency and allowing for verification of the generated content.
Multimodal Retrieval Augmented Generation (MM-RAG)
.jpg)
The Need for Multimodal Knowledge Retrieval
While RAG has proven to be a powerful technique for enhancing language models, it primarily focuses on textual knowledge sources. However, our world is inherently multimodal, with information and knowledge in various formats, including images, videos, and audio recordings.
To comprehensively capture and represent the richness of human knowledge and experiences, AI systems must be capable of processing and integrating information from multiple modalities. This need has led to the development of Multimodal Retrieval Augmented Generation, an extension of the RAG framework incorporating multimodal knowledge retrieval.
What is MM-RAG?
MM-RAG is a technique that combines multimodal pre-trained models, such as large multimodal models (LMMs), with the ability to retrieve and integrate relevant multimodal information from external knowledge sources. MM-RAG extends the RAG framework by enabling AI systems to access and leverage knowledge from various modalities, including text, images, videos, and audio recordings.
By incorporating multimodal knowledge, MM-RAG aims to enhance the performance of generative AI systems in tasks that require a comprehensive understanding of the world and the ability to reason across multiple modalities. This approach offers several advantages over traditional text-only RAG, including improved coherence, relevance, and accuracy in generated outputs and the ability to handle a broader range of tasks and scenarios.
How MM-RAG Works
Pre-training on Multimodal Data
The foundation of MM-RAG lies in the pre-training of multimodal models on massive datasets containing various modalities, such as image-text, video-text, and audio-text pairs. These pre-trained models, known as large multimodal models (LMMs), learn to map and align concepts across different modalities, creating a shared multimodal embedding space.
This pre-training process enables LMMs to develop a profound understanding of the relationships and connections between different modalities, allowing them to process and reason about multimodal information effectively.
Retrieval of Relevant Multimodal Context
In the MM-RAG framework, the retrieval component identifies and retrieves relevant multimodal context from external knowledge sources based on the input prompt or query. This involves leveraging advanced multimodal search and retrieval techniques, such as multimodal embeddings and similarity search algorithms.
By representing different modalities (text, images, videos, audio) in a shared embedding space, MM-RAG can perform cross-modal retrieval, where relevant information from any modality can be retrieved based on the input query, regardless of its modality.
Augmented Generation with Multimodal Context
Once the relevant multimodal context has been retrieved, it is incorporated into the LMM's generation process. The retrieved multimodal information serves as additional context, guiding and informing the generation of coherent, relevant, and accurate outputs across multiple modalities.
For example, in a task such as visual question answering, MM-RAG can retrieve relevant images, text descriptions, and other multimodal information to generate a comprehensive and grounded answer to the given question.
Applications of MM-RAG
Visual Question Answering
One essential application of MM-RAG is visual question answering, where the system is tasked with answering questions based on visual inputs, such as images or videos. Using multimodal knowledge retrieval and generation, MM-RAG can provide accurate and contextually relevant answers by considering textual and visual information.
Multimodal Summarization
MM-RAG can also be applied to multimodal summarization tasks, where the system needs to generate concise summaries by integrating information from various modalities, such as text documents, images, and videos. This is particularly useful in domains like news reporting, where information is often presented in a multimodal format.
Multimodal Dialogue Systems
In conversational AI, MM-RAG can enhance dialogue systems' capabilities by enabling them to understand and respond to multimodal inputs, such as text messages accompanied by images or videos. This can lead to more realistic and exciting conversations and improved task completion rates in various domains.
Text-to-Image Generation
MM-RAG can also facilitate text-to-image generation tasks, where the system generates relevant and contextually appropriate images based on textual descriptions or prompts. This has applications in creative design, advertising, and visual storytelling.
Video Understanding and Generation
Another exciting application of MM-RAG is video understanding and generation. The system can process and comprehend video content and generate new video clips based on multimodal inputs or prompts. This could affect video editing, content creation, and multimedia entertainment.
Challenges and Future Directions
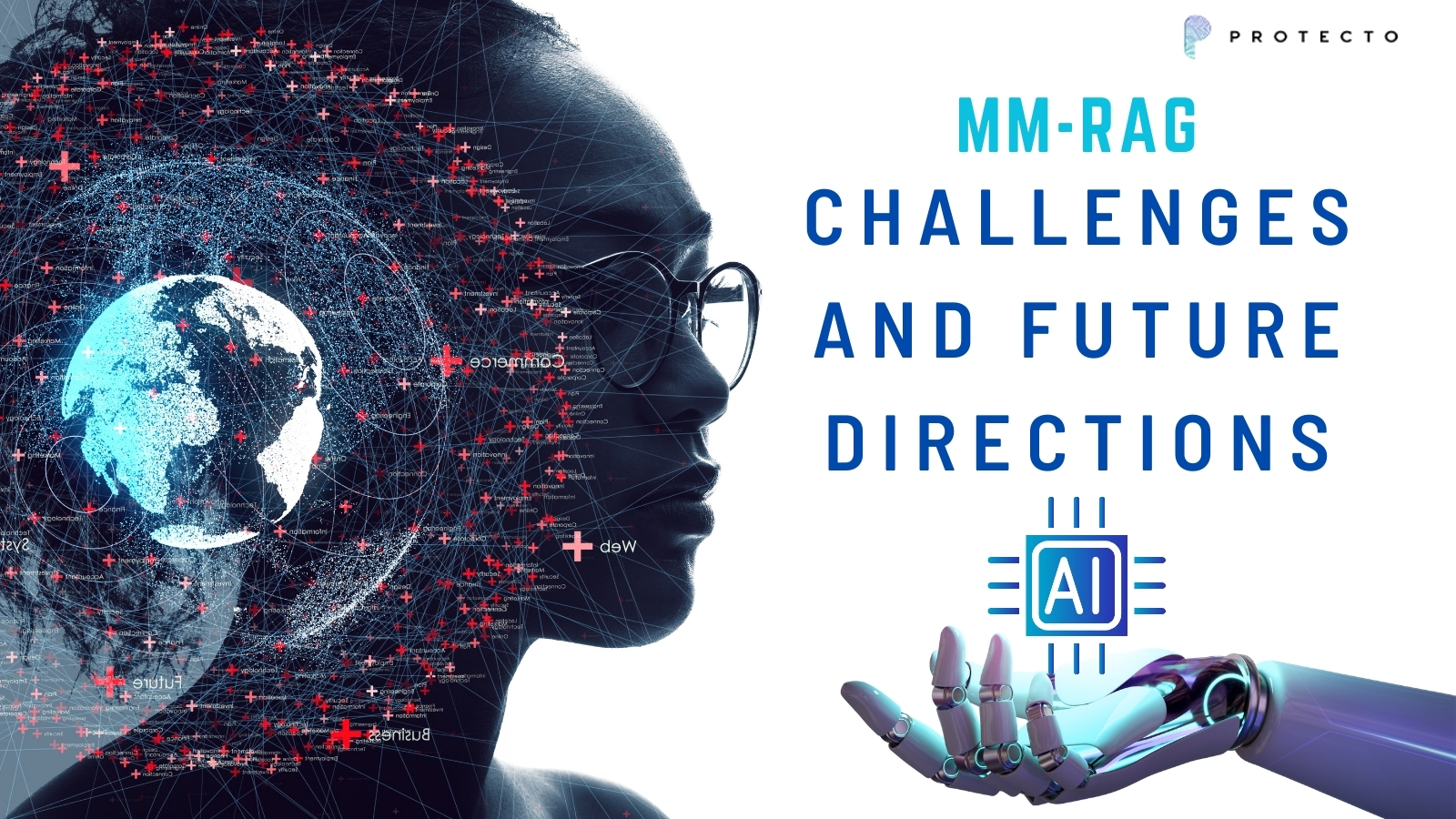
Scalability and Computational Cost
One of the significant challenges in implementing MM-RAG is scalability and computational cost. Multimodal models and multimodal knowledge retrieval require substantial computational resources and storage capacity, mainly when dealing with large-scale datasets and multiple modalities.
Multimodal Data Availability and Quality
The performance of MM-RAG heavily relies on the availability and quality of multimodal data used for pre-training and knowledge retrieval. However, curating and maintaining high-quality multimodal datasets can be challenging and resource-intensive, particularly for specialized domains or uncommon modalities.
Evaluation Metrics for Multimodal Generation
Evaluating the performance of multimodal generative models is a complex task, as it requires assessing the quality, coherence, and accuracy of outputs across multiple modalities. Existing evaluation metrics and benchmarks may not be sufficient to capture the nuances and intricacies of multimodal generation tasks.
Ethical Considerations and Potential Biases
As with any AI system, MM-RAG models and applications must be developed and deployed with careful consideration of ethical implications and potential biases. Data privacy, responsible data collection, and mitigating biases in multimodal data and models should be addressed proactively.
To ensure the responsible and ethical development of MM-RAG technologies, ongoing research, and collaboration between AI researchers, domain experts, and ethics committees will be essential.
Final Thoughts
Multimodal Retrieval Augmented Generation represents a significant leap forward in AI, unlocking the potential to develop systems that can understand, reason, and generate content across multiple modalities. By combining the power of multimodal pre-training, cross-modal knowledge retrieval, and language generation, MM-RAG offers a promising path towards more human-like AI systems capable of processing and integrating information from the rich tapestry of modalities that make up our world.
As research in this area continues to advance, MM-RAG has the potential to revolutionize various industries and applications, from creative design and content creation to education, healthcare, and beyond. MM-RAG opens up new frontiers in human-computer interaction and intelligent decision-making by enabling AI systems to seamlessly integrate textual, visual, auditory, and other forms of information.
Embracing the Multimodal Future
As we tackle this compelling journey towards multimodal AI, researchers, developers, and industry leaders must embrace the opportunities and challenges that lie ahead. Collaboration between experts from various fields, such as computer vision, natural language processing, and machine learning, will be instrumental in driving innovation and overcoming the technical and ethical hurdles that arise.
By fostering interdisciplinary efforts and investing in the development of MM-RAG technologies, we can unlock the full potential of multimodal AI, paving the way for a future where intelligent systems can genuinely understand and interact with the world in all its multifaceted complexity.